Proteomics
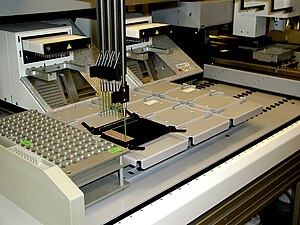
Proteomics is the large-scale study of proteins.[1][2] Proteins are vital macromolecules of all living organisms, with many functions such as the formation of structural fibers of muscle tissue, enzymatic digestion of food, or synthesis and replication of DNA. In addition, other kinds of proteins include antibodies that protect an organism from infection, and hormones that send important signals throughout the body.
The proteome is the entire set of proteins produced or modified by an organism or system. Proteomics enables the identification of ever-increasing numbers of proteins. This varies with time and distinct requirements, or stresses, that a cell or organism undergoes.[3]
Proteomics is an interdisciplinary domain that has benefited greatly from the genetic information of various genome projects, including the Human Genome Project.[4] It covers the exploration of proteomes from the overall level of protein composition, structure, and activity, and is an important component of functional genomics.
Proteomics generally denotes the large-scale experimental analysis of proteins and proteomes, but often refers specifically to protein purification and mass spectrometry. Indeed, mass spectrometry is the most powerful method for analysis of proteomes, both in large samples composed of millions of cells[5] and in single cells.[6][7]
History and etymology
[edit]The first studies of proteins that could be regarded as proteomics began in 1974, after the introduction of the two-dimensional gel and mapping of the proteins from the bacterium Escherichia coli.[8]
Proteome is a blend of the words "protein" and "genome". It was coined in 1994 by then-Ph.D student Marc Wilkins at Macquarie University,[9] which founded the first dedicated proteomics laboratory in 1995.[10][11]
Complexity of the problem
[edit]After genomics and transcriptomics, proteomics is the next step in the study of biological systems. It is more complicated than genomics because an organism's genome is more or less constant, whereas proteomes differ from cell to cell and from time to time. Distinct genes are expressed in different cell types, which means that even the basic set of proteins produced in a cell must be identified.[citation needed]
In the past this phenomenon was assessed by RNA analysis, which was found to lack correlation with protein content.[12][13] It is now known that mRNA is not always translated into protein,[14] and the amount of protein produced for a given amount of mRNA depends on the gene it is transcribed from and on the cell's physiological state. Proteomics confirms the presence of the protein and provides a direct measure of its quantity.[citation needed]
Post-translational modifications
[edit]Not only does the translation from mRNA cause differences, but many proteins also are subjected to a wide variety of chemical modifications after translation. The most common and widely studied post-translational modifications include phosphorylation and glycosylation. Many of these post-translational modifications are critical to the protein's function.[citation needed]
Phosphorylation
[edit]One such modification is phosphorylation, which happens to many enzymes and structural proteins in the process of cell signaling. The addition of a phosphate to particular amino acids—most commonly serine and threonine[15] mediated by serine-threonine kinases, or more rarely tyrosine mediated by tyrosine kinases—causes a protein to become a target for binding or interacting with a distinct set of other proteins that recognize the phosphorylated domain.[citation needed]
Because protein phosphorylation is one of the most studied protein modifications, many "proteomic" efforts are geared to determining the set of phosphorylated proteins in a particular cell or tissue-type under particular circumstances. This alerts the scientist to the signaling pathways that may be active in that instance.
Ubiquitination
[edit]Ubiquitin is a small protein that may be affixed to certain protein substrates by enzymes called E3 ubiquitin ligases. Determining which proteins are poly-ubiquitinated helps understand how protein pathways are regulated. This is, therefore, an additional legitimate "proteomic" study. Similarly, once a researcher determines which substrates are ubiquitinated by each ligase, determining the set of ligases expressed in a particular cell type is helpful.[citation needed]
Additional modifications
[edit]In addition to phosphorylation and ubiquitination, proteins may be subjected to (among others) methylation, acetylation, glycosylation, oxidation, and nitrosylation. Some proteins undergo all these modifications, often in time-dependent combinations. This illustrates the potential complexity of studying protein structure and function.
Distinct proteins are made under distinct settings
[edit]A cell may make different sets of proteins at different times or under different conditions, for example during development, cellular differentiation, cell cycle, or carcinogenesis. Further increasing proteome complexity, as mentioned, most proteins are able to undergo a wide range of post-translational modifications.
Therefore, a "proteomics" study may become complex very quickly, even if the topic of study is restricted. In more ambitious settings, such as when a biomarker for a specific cancer subtype is sought, the proteomics scientist might elect to study multiple blood serum samples from multiple cancer patients to minimise confounding factors and account for experimental noise.[16] Thus, complicated experimental designs are sometimes necessary to account for the dynamic complexity of the proteome.
Limitations of genomics and proteomics studies
[edit]Proteomics gives a different level of understanding than genomics for many reasons:
- the level of transcription of a gene gives only a rough estimate of its level of translation into a protein.[17] An mRNA produced in abundance may be degraded rapidly or translated inefficiently, resulting in a small amount of protein.
- as mentioned above, many proteins experience post-translational modifications that profoundly affect their activities; for example, some proteins are not active until they become phosphorylated. Methods such as phosphoproteomics and glycoproteomics are used to study post-translational modifications.
- many transcripts give rise to more than one protein, through alternative splicing or alternative post-translational modifications.
- many proteins form complexes with other proteins or RNA molecules, and only function in the presence of these other molecules.
- protein degradation rate plays an important role in protein content.[18]
Reproducibility. One major factor affecting reproducibility in proteomics experiments is the simultaneous elution of many more peptides than mass spectrometers can measure. This causes stochastic differences between experiments due to data-dependent acquisition of tryptic peptides. Although early large-scale shotgun proteomics analyses showed considerable variability between laboratories,[19][20] presumably due in part to technical and experimental differences between laboratories, reproducibility has been improved in more recent mass spectrometry analysis, particularly on the protein level.[21] Notably, targeted proteomics shows increased reproducibility and repeatability compared with shotgun methods, although at the expense of data density and effectiveness.[22]
Data quality. Proteomic analysis is highly amenable to automation and large data sets are created, which are processed by software algorithms. Filter parameters are used to reduce the number of false hits, but they cannot be completely eliminated. Scientists have expressed the need for awareness that proteomics experiments should adhere to the criteria of analytical chemistry (sufficient data quality, sanity check, validation).[23][24][25][26]
Methods of studying proteins
[edit]In proteomics, there are multiple methods to study proteins. Generally, proteins may be detected by using either antibodies (immunoassays), electrophoretic separation or mass spectrometry. If a complex biological sample is analyzed, either a very specific antibody needs to be used in quantitative dot blot analysis (QDB), or biochemical separation then needs to be used before the detection step, as there are too many analytes in the sample to perform accurate detection and quantification.
Protein detection with antibodies (immunoassays)
[edit]Antibodies to particular proteins, or their modified forms, have been used in biochemistry and cell biology studies. These are among the most common tools used by molecular biologists today. There are several specific techniques and protocols that use antibodies for protein detection. The enzyme-linked immunosorbent assay (ELISA) has been used for decades to detect and quantitatively measure proteins in samples. The western blot may be used for detection and quantification of individual proteins, where in an initial step, a complex protein mixture is separated using SDS-PAGE and then the protein of interest is identified using an antibody.[citation needed]
Modified proteins may be studied by developing an antibody specific to that modification. For example, some antibodies only recognize certain proteins when they are tyrosine-phosphorylated, they are known as phospho-specific antibodies. Also, there are antibodies specific to other modifications. These may be used to determine the set of proteins that have undergone the modification of interest.[citation needed]
Immunoassays can also be carried out using recombinantly generated immunoglobulin derivatives or synthetically designed protein scaffolds that are selected for high antigen specificity. Such binders include single domain antibody fragments (Nanobodies),[27] designed ankyrin repeat proteins (DARPins)[28] and aptamers.[29]
Disease detection at the molecular level is driving the emerging revolution of early diagnosis and treatment. A challenge facing the field is that protein biomarkers for early diagnosis may be present in very low abundance. The lower limit of detection with conventional immunoassay technology is the upper femtomolar range (10−13 M). Digital immunoassay technology has improved detection sensitivity three logs, to the attomolar range (10−16 M). This capability has the potential to open new advances in diagnostics and therapeutics, but such technologies have been relegated to manual procedures that are not well suited for efficient routine use.[30]
Antibody-free protein detection
[edit]While protein detection with antibodies is still very common in molecular biology, other methods have been developed as well, that do not rely on an antibody. These methods offer various advantages, for instance they often are able to determine the sequence of a protein or peptide, they may have higher throughput than antibody-based, and they sometimes can identify and quantify proteins for which no antibody exists.
Detection methods
[edit]One of the earliest methods for protein analysis has been Edman degradation (introduced in 1967) where a single peptide is subjected to multiple steps of chemical degradation to resolve its sequence. These early methods have mostly been supplanted by technologies that offer higher throughput.[citation needed]
More recently implemented methods use mass spectrometry-based techniques, a development that was made possible by the discovery of "soft ionization" methods developed in the 1980s, such as matrix-assisted laser desorption/ionization (MALDI) and electrospray ionization (ESI). These methods gave rise to the top-down and the bottom-up proteomics workflows where often additional separation is performed before analysis (see below).
Separation methods
[edit]For the analysis of complex biological samples, a reduction of sample complexity is required. This may be performed off-line by one-dimensional or two-dimensional separation. More recently, on-line methods have been developed where individual peptides (in bottom-up proteomics approaches) are separated using reversed-phase chromatography and then, directly ionized using ESI; the direct coupling of separation and analysis explains the term "on-line" analysis.
Hybrid technologies
[edit]Several hybrid technologies use antibody-based purification of individual analytes and then perform mass spectrometric analysis for identification and quantification. Examples of these methods are the MSIA (mass spectrometric immunoassay), developed by Randall Nelson in 1995,[31] and the SISCAPA (Stable Isotope Standard Capture with Anti-Peptide Antibodies) method, introduced by Leigh Anderson in 2004.[32]
Current research methodologies
[edit]Fluorescence two-dimensional differential gel electrophoresis (2-D DIGE)[33] may be used to quantify variation in the 2-D DIGE process and establish statistically valid thresholds for assigning quantitative changes between samples.[33]
Comparative proteomic analysis may reveal the role of proteins in complex biological systems, including reproduction. For example, treatment with the insecticide triazophos causes an increase in the content of brown planthopper (Nilaparvata lugens (Stål)) male accessory gland proteins (Acps) that may be transferred to females via mating, causing an increase in fecundity (i.e. birth rate) of females.[34] To identify changes in the types of accessory gland proteins (Acps) and reproductive proteins that mated female planthoppers received from male planthoppers, researchers conducted a comparative proteomic analysis of mated N. lugens females.[35] The results indicated that these proteins participate in the reproductive process of N. lugens adult females and males.[35]
Proteome analysis of Arabidopsis peroxisomes[36] has been established as the major unbiased approach for identifying new peroxisomal proteins on a large scale.[36]
There are many approaches to characterizing the human proteome, which is estimated to contain between 20,000 and 25,000 non-redundant proteins. The number of unique protein species likely will increase by between 50,000 and 500,000 due to RNA splicing and proteolysis events, and when post-translational modification also are considered, the total number of unique human proteins is estimated to range in the low millions.[37][38]
In addition, the first promising attempts to decipher the proteome of animal tumors have recently been reported.[39] This method was used as a functional method in Macrobrachium rosenbergii protein profiling.[40]
High-throughput proteomic technologies
[edit]Proteomics has steadily gained momentum over the past decade with the evolution of several approaches. Few of these are new, and others build on traditional methods. Mass spectrometry-based methods, affinity proteomics, and micro arrays are the most common technologies for large-scale study of proteins.
Mass spectrometry and protein profiling
[edit]
There are two mass spectrometry-based methods currently used for protein profiling. The more established and widespread method uses high resolution, two-dimensional electrophoresis to separate proteins from different samples in parallel, followed by selection and staining of differentially expressed proteins to be identified by mass spectrometry. Despite the advances in 2-DE and its maturity, it has its limits as well. The central concern is the inability to resolve all the proteins within a sample, given their dramatic range in expression level and differing properties. The combination of pore size, and protein charge, size and shape can greatly determine migration rate which leads to other complications.[41]
The second quantitative approach uses stable isotope tags to differentially label proteins from two different complex mixtures.[42][43] Here, the proteins within a complex mixture are labeled isotopically first, and then digested to yield labeled peptides. The labeled mixtures are then combined, the peptides separated by multidimensional liquid chromatography and analyzed by tandem mass spectrometry. Isotope coded affinity tag (ICAT) reagents are the widely used isotope tags. In this method, the cysteine residues of proteins get covalently attached to the ICAT reagent, thereby reducing the complexity of the mixtures omitting the non-cysteine residues.
Quantitative proteomics using stable isotopic tagging is an increasingly useful tool in modern development. Firstly, chemical reactions have been used to introduce tags into specific sites or proteins for the purpose of probing specific protein functionalities. The isolation of phosphorylated peptides has been achieved using isotopic labeling and selective chemistries to capture the fraction of protein among the complex mixture. Secondly, the ICAT technology was used to differentiate between partially purified or purified macromolecular complexes such as large RNA polymerase II pre-initiation complex and the proteins complexed with yeast transcription factor. Thirdly, ICAT labeling was recently combined with chromatin isolation to identify and quantify chromatin-associated proteins. Finally ICAT reagents are useful for proteomic profiling of cellular organelles and specific cellular fractions.[41]
Another quantitative approach is the accurate mass and time (AMT) tag approach developed by Richard D. Smith and coworkers at Pacific Northwest National Laboratory. In this approach, increased throughput and sensitivity is achieved by avoiding the need for tandem mass spectrometry, and making use of precisely determined separation time information and highly accurate mass determinations for peptide and protein identifications.
Affinity proteomics
[edit]Affinity proteomics uses antibodies or other affinity reagents (such as oligonucleotide-based aptamers) as protein-specific detection probes.[44] Currently this method can interrogate several thousand proteins, typically from biofluids such as plasma, serum or cerebrospinal fluid (CSF). A key differentiator for this technology is the ability to analyze hundreds or thousands of samples in a reasonable timeframe (a matter of days or weeks); mass spectrometry-based methods are not scalable to this level of sample throughput for proteomics analyses.
Protein chips
[edit]Balancing the use of mass spectrometers in proteomics and in medicine is the use of protein micro arrays. The aim behind protein micro arrays is to print thousands of protein detecting features for the interrogation of biological samples. Antibody arrays are an example in which a host of different antibodies are arrayed to detect their respective antigens from a sample of human blood. Another approach is the arraying of multiple protein types for the study of properties like protein-DNA, protein-protein and protein-ligand interactions. Ideally, the functional proteomic arrays would contain the entire complement of the proteins of a given organism. The first version of such arrays consisted of 5000 purified proteins from yeast deposited onto glass microscopic slides. Despite the success of first chip, it was a greater challenge for protein arrays to be implemented. Proteins are inherently much more difficult to work with than DNA. They have a broad dynamic range, are less stable than DNA and their structure is difficult to preserve on glass slides, though they are essential for most assays. The global ICAT technology has striking advantages over protein chip technologies.[41]
Reverse-phased protein microarrays
[edit]
This is a promising and newer microarray application for the diagnosis, study and treatment of complex diseases such as cancer. The technology merges laser capture microdissection (LCM) with micro array technology, to produce reverse-phase protein microarrays. In this type of microarrays, the whole collection of protein themselves are immobilized with the intent of capturing various stages of disease within an individual patient. When used with LCM, reverse phase arrays can monitor the fluctuating state of proteome among different cell population within a small area of human tissue. This is useful for profiling the status of cellular signaling molecules, among a cross-section of tissue that includes both normal and cancerous cells. This approach is useful in monitoring the status of key factors in normal prostate epithelium and invasive prostate cancer tissues. LCM then dissects these tissue and protein lysates were arrayed onto nitrocellulose slides, which were probed with specific antibodies. This method can track all kinds of molecular events and can compare diseased and healthy tissues within the same patient enabling the development of treatment strategies and diagnosis. The ability to acquire proteomics snapshots of neighboring cell populations, using reverse-phase microarrays in conjunction with LCM has a number of applications beyond the study of tumors. The approach can provide insights into normal physiology and pathology of all the tissues and is invaluable for characterizing developmental processes and anomalies.[41]
Protein Detection via Bioorthogonal Chemistry
[edit]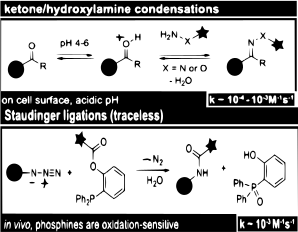
Recent advancements in bioorthogonal chemistry have revealed applications in protein analysis. The extension of using organic molecules to observe their reaction with proteins reveals extensive methods to tag them. Unnatural amino acids and various functional groups represent new growing technologies in proteomics.
Specific biomolecules that are capable of being metabolized in cells or tissues are inserted into proteins or glycans. The molecule will have an affinity tag, modifying the protein allowing it to be detected. Azidohomoalanine (AHA) utilizes this affinity tag via incorporation with Met-t-RNA synthetase to incorporate into proteins. This has allowed AHA to assist in determine the identity of newly synthesized proteins created in response to perturbations and to identify proteins secreted by cells.[45]
Recent studies[46] using ketones and aldehydes condensations show that they are best suited for in vitro or cell surface labeling. However, using ketones and aldehydes as bioorthogonal reporters revealed slow kinetics indicating that while effective for labeling, the concentration must be high.
Certain proteins can be detected via their reactivity to azide groups. Non-proteinogenic amino acids can bear azide groups which react with phosphines in Staudinger ligations. This reaction has already been used to label other biomolecules in living cells and animals.[47]
The bioorthoganal field is expanding and is driving further applications within proteomics. It is worthwhile noting the limitations and benefits. Rapid reactions can create bioconjuctions and create high concentrations with low amounts of reactants. Contrarily slow kinetic reactions like aldehyde and ketone condensation while effective require a high concentration making it cost inefficient.
Practical applications
[edit]New drug discovery
[edit]One major development to come from the study of human genes and proteins has been the identification of potential new drugs for the treatment of disease. This relies on genome and proteome information to identify proteins associated with a disease, which computer software can then use as targets for new drugs. For example, if a certain protein is implicated in a disease, its 3D structure provides the information to design drugs to interfere with the action of the protein. A molecule that fits the active site of an enzyme, but cannot be released by the enzyme, inactivates the enzyme. This is the basis of new drug-discovery tools, which aim to find new drugs to inactivate proteins involved in disease. As genetic differences among individuals are found, researchers expect to use these techniques to develop personalized drugs that are more effective for the individual.[48]
Proteomics is also used to reveal complex plant-insect interactions that help identify candidate genes involved in the defensive response of plants to herbivory.[49][50][51]
A branch of proteomics called chemoproteomics provides numerous tools and techniques to detect protein targets of drugs.[52]
Interaction proteomics and protein networks
[edit]Interaction proteomics is the analysis of protein interactions from scales of binary interactions to proteome- or network-wide. Most proteins function via protein–protein interactions, and one goal of interaction proteomics is to identify binary protein interactions, protein complexes, and interactomes.
Several methods are available to probe protein–protein interactions. While the most traditional method is yeast two-hybrid analysis, a powerful emerging method is affinity purification followed by protein mass spectrometry using tagged protein baits. Other methods include surface plasmon resonance (SPR),[53][54] protein microarrays, dual polarisation interferometry, microscale thermophoresis, kinetic exclusion assay, and experimental methods such as phage display and in silico computational methods.
Knowledge of protein-protein interactions is especially useful in regard to biological networks and systems biology, for example in cell signaling cascades and gene regulatory networks (GRNs, where knowledge of protein-DNA interactions is also informative). Proteome-wide analysis of protein interactions, and integration of these interaction patterns into larger biological networks, is crucial towards understanding systems-level biology.[55][56]
Expression proteomics
[edit]Expression proteomics includes the analysis of protein expression at a larger scale. It helps identify main proteins in a particular sample, and those proteins differentially expressed in related samples—such as diseased vs. healthy tissue. If a protein is found only in a diseased sample then it can be a useful drug target or diagnostic marker. Proteins with the same or similar expression profiles may also be functionally related. There are technologies such as 2D-PAGE and mass spectrometry that are used in expression proteomics.[57]
Biomarkers
[edit]The National Institutes of Health has defined a biomarker as "a characteristic that is objectively measured and evaluated as an indicator of normal biological processes, pathogenic processes, or pharmacologic responses to a therapeutic intervention."[58][59]
Understanding the proteome, the structure and function of each protein and the complexities of protein–protein interactions are critical for developing the most effective diagnostic techniques and disease treatments in the future. For example, proteomics is highly useful in the identification of candidate biomarkers (proteins in body fluids that are of value for diagnosis), identification of the bacterial antigens that are targeted by the immune response, and identification of possible immunohistochemistry markers of infectious or neoplastic diseases.[60]
An interesting use of proteomics is using specific protein biomarkers to diagnose disease. A number of techniques allow to test for proteins produced during a particular disease, which helps to diagnose the disease quickly. Techniques include western blot, immunohistochemical staining, enzyme linked immunosorbent assay (ELISA) or mass spectrometry.[39][61] Secretomics, a subfield of proteomics that studies secreted proteins and secretion pathways using proteomic approaches, has recently emerged as an important tool for the discovery of biomarkers of disease.[62]
Proteogenomics
[edit]In proteogenomics, proteomic technologies such as mass spectrometry are used for improving gene annotations. Parallel analysis of the genome and the proteome facilitates discovery of post-translational modifications and proteolytic events,[63] especially when comparing multiple species (comparative proteogenomics).[64]
Structural proteomics
[edit]Structural proteomics includes the analysis of protein structures at large-scale. It compares protein structures and helps identify functions of newly discovered genes. The structural analysis also helps to understand that where drugs bind to proteins and also shows where proteins interact with each other. This understanding is achieved using different technologies such as X-ray crystallography and NMR spectroscopy.[57]
Bioinformatics for proteomics (proteome informatics)
[edit]Much proteomics data is collected with the help of high throughput technologies such as mass spectrometry and microarray. It would often take weeks or months to analyze the data and perform comparisons by hand. For this reason, biologists and chemists are collaborating with computer scientists and mathematicians to create programs and pipeline to computationally analyze the protein data. Using bioinformatics techniques, researchers are capable of faster analysis and data storage. A good place to find lists of current programs and databases is on the ExPASy bioinformatics resource portal. The applications of bioinformatics-based proteomics include medicine, disease diagnosis, biomarker identification, and many more.
Protein identification
[edit]Mass spectrometry and microarray produce peptide fragmentation information but do not give identification of specific proteins present in the original sample. Due to the lack of specific protein identification, past researchers were forced to decipher the peptide fragments themselves. However, there are currently programs available for protein identification. These programs take the peptide sequences output from mass spectrometry and microarray and return information about matching or similar proteins. This is done through algorithms implemented by the program which perform alignments with proteins from known databases such as UniProt[65] and PROSITE[66] to predict what proteins are in the sample with a degree of certainty.
Protein structure
[edit]The biomolecular structure forms the 3D configuration of the protein. Understanding the protein's structure aids in the identification of the protein's interactions and function. It used to be that the 3D structure of proteins could only be determined using X-ray crystallography and NMR spectroscopy. As of 2017, Cryo-electron microscopy is a leading technique, solving difficulties with crystallization (in X-ray crystallography) and conformational ambiguity (in NMR); resolution was 2.2Å as of 2015. Now, through bioinformatics, there are computer programs that can in some cases predict and model the structure of proteins. These programs use the chemical properties of amino acids and structural properties of known proteins to predict the 3D model of sample proteins. This also allows scientists to model protein interactions on a larger scale. In addition, biomedical engineers are developing methods to factor in the flexibility of protein structures to make comparisons and predictions.[67]
Post-translational modifications
[edit]Most programs available for protein analysis are not written for proteins that have undergone post-translational modifications.[68] Some programs will accept post-translational modifications to aid in protein identification but then ignore the modification during further protein analysis. It is important to account for these modifications since they can affect the protein's structure. In turn, computational analysis of post-translational modifications has gained the attention of the scientific community. The current post-translational modification programs are only predictive.[69] Chemists, biologists and computer scientists are working together to create and introduce new pipelines that allow for analysis of post-translational modifications that have been experimentally identified for their effect on the protein's structure and function.
Computational methods in studying protein biomarkers
[edit]One example of the use of bioinformatics and the use of computational methods is the study of protein biomarkers. Computational predictive models[70] have shown that extensive and diverse feto-maternal protein trafficking occurs during pregnancy and can be readily detected non-invasively in maternal whole blood. This computational approach circumvented a major limitation, the abundance of maternal proteins interfering with the detection of fetal proteins, to fetal proteomic analysis of maternal blood. Computational models can use fetal gene transcripts previously identified in maternal whole blood to create a comprehensive proteomic network of the term neonate. Such work shows that the fetal proteins detected in pregnant woman's blood originate from a diverse group of tissues and organs from the developing fetus. The proteomic networks contain many biomarkers that are proxies for development and illustrate the potential clinical application of this technology as a way to monitor normal and abnormal fetal development.
An information-theoretic framework has also been introduced for biomarker discovery, integrating biofluid and tissue information.[71] This new approach takes advantage of functional synergy between certain biofluids and tissues with the potential for clinically significant findings not possible if tissues and biofluids were considered individually. By conceptualizing tissue-biofluid as information channels, significant biofluid proxies can be identified and then used for the guided development of clinical diagnostics. Candidate biomarkers are then predicted based on information transfer criteria across the tissue-biofluid channels. Significant biofluid-tissue relationships can be used to prioritize clinical validation of biomarkers.[71]
Emerging trends
[edit]A number of emerging concepts have the potential to improve the current features of proteomics. Obtaining absolute quantification of proteins and monitoring post-translational modifications are the two tasks that impact the understanding of protein function in healthy and diseased cells. Further, the throughput and sensitivity of proteomic assays, often measured as samples analyzed per day and depth of proteome coverage, respectively, have driven development of cutting-edge instrumentation and methodologies.[72] For many cellular events, the protein concentrations do not change; rather, their function is modulated by post-translational modifications (PTM). Methods of monitoring PTM are an underdeveloped area in proteomics. Selecting a particular subset of protein for analysis substantially reduces protein complexity, making it advantageous for diagnostic purposes where blood is the starting material. Another important aspect of proteomics, yet not addressed, is that proteomics methods should focus on studying proteins in the context of the environment. The increasing use of chemical cross-linkers, introduced into living cells to fix protein-protein, protein-DNA and other interactions, may ameliorate this problem partially. The challenge is to identify suitable methods of preserving relevant interactions. Another goal for studying proteins is development of more sophisticated methods to image proteins and other molecules in living cells and real-time.[41]
Systems biology
[edit]Advances in quantitative proteomics would clearly enable more in-depth analysis of cellular systems.[55][56] Another research frontier is the analysis of single cells,[73][74] and protein covariation across single cells[75] which reflects biological processes such as protein complex formation, immune functions,[76] as well as cell cycle and priming of cancer cells for drug resistance[77] Biological systems are subject to a variety of perturbations (cell cycle, cellular differentiation, carcinogenesis, environment (biophysical), etc.). Transcriptional and translational responses to these perturbations results in functional changes to the proteome implicated in response to the stimulus. Therefore, describing and quantifying proteome-wide changes in protein abundance is crucial towards understanding biological phenomenon more holistically, on the level of the entire system. In this way, proteomics can be seen as complementary to genomics, transcriptomics, epigenomics, metabolomics, and other -omics approaches in integrative analyses attempting to define biological phenotypes more comprehensively. As an example, The Cancer Proteome Atlas provides quantitative protein expression data for ~200 proteins in over 4,000 tumor samples with matched transcriptomic and genomic data from The Cancer Genome Atlas.[78] Similar datasets in other cell types, tissue types, and species, particularly using deep shotgun mass spectrometry, will be an immensely important resource for research in fields like cancer biology, developmental and stem cell biology, medicine, and evolutionary biology.
Human plasma proteome
[edit]Characterizing the human plasma proteome has become a major goal in the proteomics arena, but it is also the most challenging proteomes of all human tissues.[79] It contains immunoglobulin, cytokines, protein hormones, and secreted proteins indicative of infection on top of resident, hemostatic proteins. It also contains tissue leakage proteins due to the blood circulation through different tissues in the body. The blood thus contains information on the physiological state of all tissues and, combined with its accessibility, makes the blood proteome invaluable for medical purposes. It is thought that characterizing the proteome of blood plasma is a daunting challenge.
The depth of the plasma proteome encompasses a dynamic range of more than 1010 between the highest abundant protein (albumin) and the lowest (some cytokines) and is thought to be one of the main challenges for proteomics.[80] Temporal and spatial dynamics further complicate the study of human plasma proteome. The turnover of some proteins is quite faster than others and the protein content of an artery may substantially vary from that of a vein. All these differences make even the simplest proteomic task of cataloging the proteome seem out of reach. To tackle this problem, priorities need to be established. Capturing the most meaningful subset of proteins among the entire proteome to generate a diagnostic tool is one such priority. Secondly, since cancer is associated with enhanced glycosylation of proteins, methods that focus on this part of proteins will also be useful. Again: multiparameter analysis best reveals a pathological state. As these technologies improve, the disease profiles should be continually related to respective gene expression changes.[41] Due to the above-mentioned problems plasma proteomics remained challenging. However, technological advancements and continuous developments seem to result in a revival of plasma proteomics as it was shown recently by a technology called plasma proteome profiling.[81] Due to such technologies researchers were able to investigate inflammation processes in mice, the heritability of plasma proteomes as well as to show the effect of such a common life style change like weight loss on the plasma proteome.[82][83][84]
Journals
[edit]Numerous journals are dedicated to the field of proteomics and related areas. Note that journals dealing with proteins are usually more focused on structure and function while proteomics journals are more focused on the large-scale analysis of whole proteomes or at least large sets of proteins. Some relevant proteomics journals are listed below (with their publishers).
- Molecular and Cellular Proteomics (ASBMB)
- Journal of Proteome Research (ACS)
- Journal of Proteomics (Elsevier)
- Proteomics (Wiley)
See also
[edit]- Activity-based proteomics
- Bottom-up proteomics
- Cytomics
- Functional genomics
- Heat stabilization
- Human proteome project
- Immunoproteomics
- List of biological databases
- List of omics topics in biology
- PEGylation
- Phosphoproteomics
- Protein production
- Proteogenomics
- Proteomic chemistry
- Secretomics
- Shotgun proteomics
- Top-down proteomics
- Systems biology
- Yeast two-hybrid system
- TCP-seq
- Glycomics
Protein databases
[edit]- Human Protein Atlas
- Human Protein Reference Database
- National Center for Biotechnology Information (NCBI)
- PeptideAtlas
- Protein Data Bank (PDB)
- Protein Information Resource (PIR)
- Proteomics Identifications Database (PRIDE)
- Proteopedia—The collaborative, 3D encyclopedia of proteins and other molecules
- Swiss-Prot
- UniProt
Research centers
[edit]References
[edit]- ^ Anderson NL, Anderson NG (August 1998). "Proteome and proteomics: new technologies, new concepts, and new words". Electrophoresis. 19 (11): 1853–1861. doi:10.1002/elps.1150191103. PMID 9740045. S2CID 28933890.
- ^ Blackstock WP, Weir MP (March 1999). "Proteomics: quantitative and physical mapping of cellular proteins". Trends in Biotechnology. 17 (3): 121–127. doi:10.1016/S0167-7799(98)01245-1. PMID 10189717.
- ^ Anderson JD, Johansson HJ, Graham CS, Vesterlund M, Pham MT, Bramlett CS, et al. (March 2016). "Comprehensive Proteomic Analysis of Mesenchymal Stem Cell Exosomes Reveals Modulation of Angiogenesis via Nuclear Factor-KappaB Signaling". Stem Cells. 34 (3): 601–613. doi:10.1002/stem.2298. PMC 5785927. PMID 26782178.
- ^ Hood L, Rowen L (2013-09-13). "The Human Genome Project: big science transforms biology and medicine". Genome Medicine. 5 (9): 79. doi:10.1186/gm483. PMC 4066586. PMID 24040834.
- ^ Zhang Y, Fonslow BR, Shan B, Baek MC, Yates JR (April 2013). "Protein analysis by shotgun/bottom-up proteomics". Chemical Reviews. 113 (4): 2343–2394. doi:10.1021/cr3003533. PMC 3751594. PMID 23438204.
- ^ Gatto L, Aebersold R, Cox J, Demichev V, Derks J, Emmott E, et al. (March 2023). "Initial recommendations for performing, benchmarking and reporting single-cell proteomics experiments". Nature Methods. 20 (3): 375–386. doi:10.1038/s41592-023-01785-3. PMC 10130941. PMID 36864200. S2CID 251018292.
- ^ Slavov N (February 2021). "Single-cell protein analysis by mass spectrometry". Current Opinion in Chemical Biology. Omics. 60: 1–9. doi:10.1016/j.cbpa.2020.04.018. PMC 7767890. PMID 32599342.
- ^ Wittmann, H. G. (1974-01-01), "[48] Two-dimensional polyacrylamide gel electrophoresis for separation of ribosomal proteins", Methods in Enzymology, Nucleic Acids and Protein Synthesis Part F, vol. 30, Academic Press, pp. 497–505, doi:10.1016/0076-6879(74)30050-x, retrieved 2024-08-28
- ^ Wasinger VC, Cordwell SJ, Cerpa-Poljak A, Yan JX, Gooley AA, Wilkins MR, et al. (July 1995). "Progress with gene-product mapping of the Mollicutes: Mycoplasma genitalium". Electrophoresis. 16 (7): 1090–1094. doi:10.1002/elps.11501601185. PMID 7498152. S2CID 9269742.
- ^ Swinbanks D (December 1995). "Government backs proteome proposal". Nature. 378 (6558): 653. Bibcode:1995Natur.378..653S. doi:10.1038/378653a0. PMID 7501000.
- ^ "APAF - The Australian Proteome Analysis Facility". www.proteome.org.au. New South Wales, Australia: Macquarie University. Retrieved 2017-02-06.
- ^ Rogers S, Girolami M, Kolch W, Waters KM, Liu T, Thrall B, Wiley HS (December 2008). "Investigating the correspondence between transcriptomic and proteomic expression profiles using coupled cluster models". Bioinformatics. 24 (24): 2894–2900. doi:10.1093/bioinformatics/btn553. PMC 4141638. PMID 18974169.
- ^ Dhingra V, Gupta M, Andacht T, Fu ZF (August 2005). "New frontiers in proteomics research: a perspective". International Journal of Pharmaceutics. 299 (1–2): 1–18. doi:10.1016/j.ijpharm.2005.04.010. PMID 15979831.
- ^ Buckingham S (May 2003). "The major world of microRNAs". Retrieved 2009-01-14.
- ^ Olsen JV, Blagoev B, Gnad F, Macek B, Kumar C, Mortensen P, Mann M (November 2006). "Global, in vivo, and site-specific phosphorylation dynamics in signaling networks". Cell. 127 (3): 635–648. doi:10.1016/j.cell.2006.09.026. PMID 17081983. S2CID 7827573.
- ^ Srinivas PR, Verma M, Zhao Y, Srivastava S (August 2002). "Proteomics for cancer biomarker discovery". Clinical Chemistry. 48 (8): 1160–1169. PMID 12142368.
- ^ Gygi SP, Rochon Y, Franza BR, Aebersold R (March 1999). "Correlation between protein and mRNA abundance in yeast". Molecular and Cellular Biology. 19 (3): 1720–1730. doi:10.1128/MCB.19.3.1720. PMC 83965. PMID 10022859.
- ^ Belle A, Tanay A, Bitincka L, Shamir R, O'Shea EK (August 2006). "Quantification of protein half-lives in the budding yeast proteome". Proceedings of the National Academy of Sciences of the United States of America. 103 (35): 13004–13009. Bibcode:2006PNAS..10313004B. doi:10.1073/pnas.0605420103. PMC 1550773. PMID 16916930.
- ^ Peng J, Elias JE, Thoreen CC, Licklider LJ, Gygi SP (2003). "Evaluation of multidimensional chromatography coupled with tandem mass spectrometry (LC/LC-MS/MS) for large-scale protein analysis: the yeast proteome". Journal of Proteome Research. 2 (1): 43–50. CiteSeerX 10.1.1.460.237. doi:10.1021/pr025556v. PMID 12643542.
- ^ Washburn MP, Wolters D, Yates JR (March 2001). "Large-scale analysis of the yeast proteome by multidimensional protein identification technology". Nature Biotechnology. 19 (3): 242–247. doi:10.1038/85686. PMID 11231557. S2CID 16796135.
- ^ Tabb DL, Vega-Montoto L, Rudnick PA, Variyath AM, Ham AJ, Bunk DM, et al. (February 2010). "Repeatability and reproducibility in proteomic identifications by liquid chromatography-tandem mass spectrometry". Journal of Proteome Research. 9 (2): 761–776. doi:10.1021/pr9006365. PMC 2818771. PMID 19921851.
- ^ Domon B, Aebersold R (July 2010). "Options and considerations when selecting a quantitative proteomics strategy". Nature Biotechnology. 28 (7): 710–721. doi:10.1038/nbt.1661. PMID 20622845. S2CID 12367142.
- ^ Dupree EJ, Jayathirtha M, Yorkey H, Mihasan M, Petre BA, Darie CC (July 2020). "A Critical Review of Bottom-Up Proteomics: The Good, the Bad, and the Future of this Field". Proteomes. 8 (3): 14. doi:10.3390/proteomes8030014. PMC 7564415. PMID 32640657.
- ^ Coorssen JR, Yergey AL (December 2015). "Proteomics Is Analytical Chemistry: Fitness-for-Purpose in the Application of Top-Down and Bottom-Up Analyses". Proteomes. 3 (4): 440–453. doi:10.3390/proteomes3040440. PMC 5217385. PMID 28248279.
- ^ König S (February 2021). "Spectral quality overrides software score-A brief tutorial on the analysis of peptide fragmentation data for mass spectrometry laymen". Journal of Mass Spectrometry. 56 (2): e4616. doi:10.1002/jms.4616. PMID 32955142. S2CID 221827335.
- ^ Duncan MW (June 2012). "Good mass spectrometry and its place in good science". Journal of Mass Spectrometry. 47 (6): 795–809. Bibcode:2012JMSp...47..795D. doi:10.1002/jms.3038. PMID 22707172.
- ^ Arbabi Ghahroudi M, Desmyter A, Wyns L, Hamers R, Muyldermans S (September 1997). "Selection and identification of single domain antibody fragments from camel heavy-chain antibodies". FEBS Letters. 414 (3): 521–526. doi:10.1016/S0014-5793(97)01062-4. PMID 9323027. S2CID 5988844.
- ^ Stumpp MT, Binz HK, Amstutz P (August 2008). "DARPins: a new generation of protein therapeutics". Drug Discovery Today. 13 (15–16): 695–701. doi:10.1016/j.drudis.2008.04.013. PMID 18621567.
- ^ Jayasena SD (September 1999). "Aptamers: an emerging class of molecules that rival antibodies in diagnostics". Clinical Chemistry. 45 (9): 1628–1650. doi:10.1093/clinchem/45.9.1628. PMID 10471678.
- ^ Wilson DH, Rissin DM, Kan CW, Fournier DR, Piech T, Campbell TG, et al. (August 2016). "The Simoa HD-1 Analyzer: A Novel Fully Automated Digital Immunoassay Analyzer with Single-Molecule Sensitivity and Multiplexing". Journal of Laboratory Automation. 21 (4): 533–547. doi:10.1177/2211068215589580. PMID 26077162.
- ^ Nelson RW, Krone JR, Bieber AL, Williams P (April 1995). "Mass spectrometric immunoassay". Analytical Chemistry. 67 (7): 1153–1158. doi:10.1021/ac00103a003. OSTI 1175578. PMID 15134097.
- ^ "SISCAPA, Stable Isotope Standard Capture with Anti-Peptide Antibodies". Broad Institute of MIT and Harvard. 2015. Archived from the original on 2015-07-15. Retrieved 2015-07-15.
- ^ a b Tonge R, Shaw J, Middleton B, Rowlinson R, Rayner S, Young J, et al. (March 2001). "Validation and development of fluorescence two-dimensional differential gel electrophoresis proteomics technology". Proteomics. 1 (3): 377–396. doi:10.1002/1615-9861(200103)1:3<377::AID-PROT377>3.0.CO;2-6. PMID 11680884. S2CID 22432028.
- ^ Wang LP, Shen J, Ge LQ, Wu JC, Yang GQ, Jahn GC (November 2010). "Insecticide-induced increase in the protein content of male accessory glands and its effect on the fecundity of females in the brown planthopper, Nilaparvata lugens Stål (Hemiptera: Delphacidae)". Crop Protection. 29 (11): 1280–5. doi:10.1016/j.cropro.2010.07.009.
- ^ a b Ge LQ, Cheng Y, Wu JC, Jahn GC (October 2011). "Proteomic analysis of insecticide triazophos-induced mating-responsive proteins of Nilaparvata lugens Stål (Hemiptera: Delphacidae)". Journal of Proteome Research. 10 (10): 4597–4612. doi:10.1021/pr200414g. PMID 21800909.
- ^ a b Reumann S (May 2011). "Toward a definition of the complete proteome of plant peroxisomes: Where experimental proteomics must be complemented by bioinformatics". Proteomics. 11 (9): 1764–1779. doi:10.1002/pmic.201000681. PMID 21472859. S2CID 20337179.
- ^ Uhlen M, Ponten F (April 2005). "Antibody-based proteomics for human tissue profiling". Molecular & Cellular Proteomics. 4 (4): 384–393. doi:10.1074/mcp.R500009-MCP200. PMID 15695805.
- ^ Jensen ON (February 2004). "Modification-specific proteomics: characterization of post-translational modifications by mass spectrometry". Current Opinion in Chemical Biology. 8 (1): 33–41. doi:10.1016/j.cbpa.2003.12.009. PMID 15036154.
- ^ a b Klopfleisch R, Klose P, Weise C, Bondzio A, Multhaup G, Einspanier R, Gruber AD (December 2010). "Proteome of metastatic canine mammary carcinomas: similarities to and differences from human breast cancer". Journal of Proteome Research. 9 (12): 6380–6391. doi:10.1021/pr100671c. PMID 20932060.
- ^ Alinejad T, Bin KQ, Vejayan J, Othman RY, Bhassu S (September 2015). "Proteomic analysis of differentially expressed protein in hemocytes of wild giant freshwater prawn Macrobrachium rosenbergii infected with infectious hypodermal and hematopoietic necrosis virus (IHHNV)". Meta Gene. 5: 55–67. doi:10.1016/j.mgene.2015.05.004. PMC 4473098. PMID 26106581.
- ^ a b c d e f Weston AD, Hood L (2004). "Systems biology, proteomics, and the future of health care: toward predictive, preventative, and personalized medicine". Journal of Proteome Research. 3 (2): 179–196. CiteSeerX 10.1.1.603.4384. doi:10.1021/pr0499693. PMID 15113093.
- ^ Rozanova, Svitlana; Barkovits, Katalin; Nikolov, Miroslav; Schmidt, Carla; Urlaub, Henning; Marcus, Katrin (2021), Marcus, Katrin; Eisenacher, Martin; Sitek, Barbara (eds.), "Quantitative Mass Spectrometry-Based Proteomics: An Overview", Quantitative Methods in Proteomics, Methods in Molecular Biology, vol. 2228, New York, NY: Springer US, pp. 85–116, doi:10.1007/978-1-0716-1024-4_8, ISBN 978-1-0716-1023-7, PMID 33950486, S2CID 233740602
- ^ Nikolov, Miroslav; Schmidt, Carla; Urlaub, Henning (2012), Marcus, Katrin (ed.), "Quantitative Mass Spectrometry-Based Proteomics: An Overview", Quantitative Methods in Proteomics, Methods in Molecular Biology, vol. 893, Totowa, NJ: Humana Press, pp. 85–100, doi:10.1007/978-1-61779-885-6_7, hdl:11858/00-001M-0000-000F-C327-D, ISBN 978-1-61779-884-9, PMID 22665296, S2CID 33009117, retrieved 2023-04-14
- ^ Stoevesandt O, Taussig MJ (August 2012). "Affinity proteomics: the role of specific binding reagents in human proteome analysis". Expert Review of Proteomics. 9 (4): 401–414. doi:10.1586/epr.12.34. PMID 22967077. S2CID 19727645.
- ^ Eichelbaum K, Winter M, Berriel Diaz M, Herzig S, Krijgsveld J (October 2012). "Selective enrichment of newly synthesized proteins for quantitative secretome analysis". Nature Biotechnology. 30 (10): 984–990. doi:10.1038/nbt.2356. PMID 23000932. S2CID 2651429.
- ^ Lang K, Chin JW (January 2014). "Bioorthogonal reactions for labeling proteins". ACS Chemical Biology. 9 (1): 16–20. doi:10.1021/cb4009292. PMID 24432752.
- ^ Devaraj NK (August 2018). "The Future of Bioorthogonal Chemistry". ACS Central Science. 4 (8): 952–959. doi:10.1021/acscentsci.8b00251. PMC 6107859. PMID 30159392.
- ^ Vaidyanathan G (March 2012). "Redefining clinical trials: the age of personalized medicine". Cell. 148 (6): 1079–1080. doi:10.1016/j.cell.2012.02.041. PMID 22424218.
- ^ Rakwal R, Komatsu S (July 2000). "Role of jasmonate in the rice (Oryza sativa L.) self-defense mechanism using proteome analysis". Electrophoresis. 21 (12): 2492–2500. doi:10.1002/1522-2683(20000701)21:12<2492::AID-ELPS2492>3.0.CO;2-2. PMID 10939463. S2CID 24979515.
- ^ Wu J, Baldwin IT (2010). "New insights into plant responses to the attack from insect herbivores". Annual Review of Genetics. 44: 1–24. doi:10.1146/annurev-genet-102209-163500. PMID 20649414.
- ^ Sangha JS, Chen YH, Kaur J, Khan W, Abduljaleel Z, Alanazi MS, et al. (February 2013). "Proteome Analysis of Rice (Oryza sativa L.) Mutants Reveals Differentially Induced Proteins during Brown Planthopper (Nilaparvata lugens) Infestation". International Journal of Molecular Sciences. 14 (2): 3921–3945. doi:10.3390/ijms14023921. PMC 3588078. PMID 23434671.
- ^ Moellering RE, Cravatt BF (January 2012). "How chemoproteomics can enable drug discovery and development". Chemistry & Biology. 19 (1): 11–22. doi:10.1016/j.chembiol.2012.01.001. PMC 3312051. PMID 22284350.
- ^ de Mol NJ (2012). "Surface Plasmon Resonance for Proteomics". Chemical Genomics and Proteomics. Methods in Molecular Biology. Vol. 800. pp. 33–53. doi:10.1007/978-1-61779-349-3_4. ISBN 978-1-61779-348-6. PMID 21964781.
- ^ Visser NF, Heck AJ (June 2008). "Surface plasmon resonance mass spectrometry in proteomics". Expert Review of Proteomics. 5 (3): 425–433. doi:10.1586/14789450.5.3.425. PMID 18532910. S2CID 11772983.
- ^ a b Bensimon A, Heck AJ, Aebersold R (7 July 2012). "Mass spectrometry-based proteomics and network biology". Annual Review of Biochemistry. 81 (1): 379–405. doi:10.1146/annurev-biochem-072909-100424. PMID 22439968.
- ^ a b Sabidó E, Selevsek N, Aebersold R (August 2012). "Mass spectrometry-based proteomics for systems biology". Current Opinion in Biotechnology. 23 (4): 591–597. doi:10.1016/j.copbio.2011.11.014. PMID 22169889.
- ^ a b "What is Proteomics?". ProteoConsult.[unreliable medical source?]
- ^ Strimbu K, Tavel JA (November 2010). "What are biomarkers?". Current Opinion in HIV and AIDS. 5 (6): 463–466. doi:10.1097/COH.0b013e32833ed177. PMC 3078627. PMID 20978388.
- ^ Biomarkers Definitions Working Group (March 2001). "Biomarkers and surrogate endpoints: preferred definitions and conceptual framework". Clinical Pharmacology and Therapeutics. 69 (3): 89–95. doi:10.1067/mcp.2001.113989. PMID 11240971. S2CID 288484.
- ^ Ceciliani F, Eckersall D, Burchmore R, Lecchi C (March 2014). "Proteomics in veterinary medicine: applications and trends in disease pathogenesis and diagnostics". Veterinary Pathology. 51 (2): 351–362. doi:10.1177/0300985813502819. hdl:2434/226049. PMID 24045891. S2CID 25693263.
- ^ Klopfleisch R, Gruber AD (May 2009). "Increased expression of BRCA2 and RAD51 in lymph node metastases of canine mammary adenocarcinomas". Veterinary Pathology. 46 (3): 416–422. doi:10.1354/vp.08-VP-0212-K-FL. PMID 19176491. S2CID 11583190.
- ^ Hathout Y (April 2007). "Approaches to the study of the cell secretome". Expert Review of Proteomics. 4 (2): 239–248. doi:10.1586/14789450.4.2.239. PMID 17425459. S2CID 26169223.
- ^ Gupta N, Tanner S, Jaitly N, Adkins JN, Lipton M, Edwards R, et al. (September 2007). "Whole proteome analysis of post-translational modifications: applications of mass-spectrometry for proteogenomic annotation". Genome Research. 17 (9): 1362–1377. doi:10.1101/gr.6427907. PMC 1950905. PMID 17690205.
- ^ Gupta N, Benhamida J, Bhargava V, Goodman D, Kain E, Kerman I, et al. (July 2008). "Comparative proteogenomics: combining mass spectrometry and comparative genomics to analyze multiple genomes". Genome Research. 18 (7): 1133–1142. doi:10.1101/gr.074344.107. PMC 2493402. PMID 18426904.
- ^ "UniProt". www.uniprot.org.
- ^ "ExPASy - PROSITE". prosite.expasy.org.
- ^ Wang HW, Chu CH, Wang WC, Pai TW (April 2014). "A local average distance descriptor for flexible protein structure comparison". BMC Bioinformatics. 15 (95): 95. doi:10.1186/1471-2105-15-95. PMC 3992163. PMID 24694083.
- ^ Petrov D, Margreitter C, Grandits M, Oostenbrink C, Zagrovic B (July 2013). "A systematic framework for molecular dynamics simulations of protein post-translational modifications". PLOS Computational Biology. 9 (7): e1003154. Bibcode:2013PLSCB...9E3154P. doi:10.1371/journal.pcbi.1003154. PMC 3715417. PMID 23874192.
- ^ Margreitter C, Petrov D, Zagrovic B (July 2013). "Vienna-PTM web server: a toolkit for MD simulations of protein post-translational modifications". Nucleic Acids Research. 41 (Web Server issue): W422–W426. doi:10.1093/nar/gkt416. PMC 3692090. PMID 23703210.
- ^ Maron JL, Alterovitz G, Ramoni M, Johnson KL, Bianchi DW (December 2009). "High-throughput discovery and characterization of fetal protein trafficking in the blood of pregnant women". Proteomics. Clinical Applications. 3 (12): 1389–1396. doi:10.1002/prca.200900109. PMC 2825712. PMID 20186258.
- ^ a b Alterovitz G, Xiang M, Liu J, Chang A, Ramoni MF (2008). "System-wide peripheral biomarker discovery using information theory". Biocomputing 2008. pp. 231–42. doi:10.1142/9789812776136_0024. ISBN 9789812776082. PMID 18229689.
{{cite book}}
:|journal=
ignored (help) - ^ Peters-Clarke, Trenton M.; Coon, Joshua J.; Riley, Nicholas M. (2024-05-21). "Instrumentation at the Leading Edge of Proteomics". Analytical Chemistry. 96 (20): 7976–8010. doi:10.1021/acs.analchem.3c04497. ISSN 0003-2700.
- ^ Slavov N (January 2022). "Scaling Up Single-Cell Proteomics". Molecular & Cellular Proteomics. 21 (1): 100179. doi:10.1016/j.mcpro.2021.100179. PMC 8683604. PMID 34808355.
- ^ Derks J, Leduc A, Wallmann G, Huffman RG, Willetts M, Khan S, et al. (July 2022). "Increasing the throughput of sensitive proteomics by plexDIA". Nature Biotechnology. 41 (1): 50–59. doi:10.1038/s41587-022-01389-w. PMC 9839897. PMID 35835881.
- ^ Slavov N (January 2022). "Learning from natural variation across the proteomes of single cells". PLOS Biology. 20 (1): e3001512. doi:10.1371/journal.pbio.3001512. PMC 8765665. PMID 34986167.
- ^ Huffman RG, Leduc A, Wichmann C, di Gioia M, Borriello F, Specht H, et al. (2022-03-18). "Prioritized single-cell proteomics reveals molecular and functional polarization across primary macrophages". bioRxiv: 2022.03.16.484655. doi:10.1101/2022.03.16.484655. S2CID 247599981.
- ^ Leduc A, Huffman RG, Cantlon J, Khan S, Slavov N (December 2022). "Exploring functional protein covariation across single cells using nPOP". Genome Biology. 23 (1): 261. doi:10.1186/s13059-022-02817-5. PMC 9756690. PMID 36527135.
- ^ Li J, Lu Y, Akbani R, Ju Z, Roebuck PL, Liu W, et al. (November 2013). "TCPA: a resource for cancer functional proteomics data". Nature Methods. 10 (11): 1046–1047. doi:10.1038/nmeth.2650. PMC 4076789. PMID 24037243.
- ^ Anderson NL (February 2010). "The clinical plasma proteome: a survey of clinical assays for proteins in plasma and serum". Clinical Chemistry. 56 (2): 177–185. doi:10.1373/clinchem.2009.126706. PMID 19884488.
- ^ Anderson L (July 2014). "Six decades searching for meaning in the proteome". Journal of Proteomics. 107: 24–30. doi:10.1016/j.jprot.2014.03.005. PMID 24642211.
- ^ Geyer PE, Kulak NA, Pichler G, Holdt LM, Teupser D, Mann M (March 2016). "Plasma Proteome Profiling to Assess Human Health and Disease". Cell Systems. 2 (3): 185–195. doi:10.1016/j.cels.2016.02.015. hdl:11858/00-001M-0000-002B-A17E-4. PMID 27135364.
- ^ Malmström E, Kilsgård O, Hauri S, Smeds E, Herwald H, Malmström L, Malmström J (January 2016). "Large-scale inference of protein tissue origin in gram-positive sepsis plasma using quantitative targeted proteomics". Nature Communications. 7: 10261. Bibcode:2016NatCo...710261M. doi:10.1038/ncomms10261. PMC 4729823. PMID 26732734.
- ^ Geyer PE, Wewer Albrechtsen NJ, Tyanova S, Grassl N, Iepsen EW, Lundgren J, et al. (December 2016). "Proteomics reveals the effects of sustained weight loss on the human plasma proteome". Molecular Systems Biology. 12 (12): 901. doi:10.15252/msb.20167357. PMC 5199119. PMID 28007936.
- ^ Liu Y, Buil A, Collins BC, Gillet LC, Blum LC, Cheng LY, et al. (February 2015). "Quantitative variability of 342 plasma proteins in a human twin population". Molecular Systems Biology. 11 (1): 786. doi:10.15252/msb.20145728. PMC 4358658. PMID 25652787.
Bibliography
[edit]- Arora PS, Yamagiwa H, Srivastava A, Bolander ME, Sarkar G (2005). "Comparative evaluation of two two-dimensional gel electrophoresis image analysis software applications using synovial fluids from patients with joint disease". Journal of Orthopaedic Science. 10 (2): 160–166. doi:10.1007/s00776-004-0878-0. PMID 15815863. S2CID 45193214.
- Belhajjame K, Embury SM, Fan H, Goble CA, Hermjakob H, Hubbard SJ, et al. (September 2005). Proteome Data Integration: Characteristics and Challenges (PDF). Proceedings of the UK e-Science All Hands Meeting. Nottingham, UK. ISBN 978-1-904425-53-3. Archived from the original (PDF) on 28 June 2006.
- Ceciliani F, Eckersall D, Burchmore R, Lecchi C (March 2014). "Proteomics in veterinary medicine: applications and trends in disease pathogenesis and diagnostics". Veterinary Pathology. 51 (2): 351–62. doi:10.1177/0300985813502819. hdl:2434/226049. PMID 24045891. S2CID 25693263.
- Liebler DC (2002). Introduction to proteomics: tools for the new biology. Totowa, NJ: Humana Press. ISBN 978-0-89603-992-6. ISBN 0-585-41879-9 (electronic, on Netlibrary?), ISBN 0-89603-991-9 hbk
- Macaulay IC, Carr P, Gusnanto A, Ouwehand WH, Fitzgerald D, Watkins NA (December 2005). "Platelet genomics and proteomics in human health and disease". The Journal of Clinical Investigation. 115 (12): 3370–3377. doi:10.1172/JCI26885. PMC 1297260. PMID 16322782.
- Naven T, Westermeier R (2002). Proteomics in Practice: A Laboratory Manual of Proteome Analysis. Weinheim: Wiley-VCH. ISBN 978-3-527-30354-0. (focused on 2D-gels, good on detail)
- Twyman RM (2004). Principles Of Proteomics (Advanced Text Series). Oxford, UK: BIOS Scientific Publishers. ISBN 978-1-85996-273-2. (covers almost all branches of proteomics)
- von Hagen J (2008). Proteomics Sample Preparation. Weinheim: Wiley-VCH. ISBN 978-3-527-31796-7.
- Wilkins MR, Williams KL, Appel RD, Hochstrasser DF (1997). Proteome Research: New Frontiers in Functional Genomics (Principles and Practice). Berlin: Springer. ISBN 978-3-540-62753-1.
- Vasan RS (May 2006). "Biomarkers of cardiovascular disease: molecular basis and practical considerations". Circulation. 113 (19): 2335–2362. doi:10.1161/CIRCULATIONAHA.104.482570. PMID 16702488.
External links
[edit]


- Proteomics at Curlie